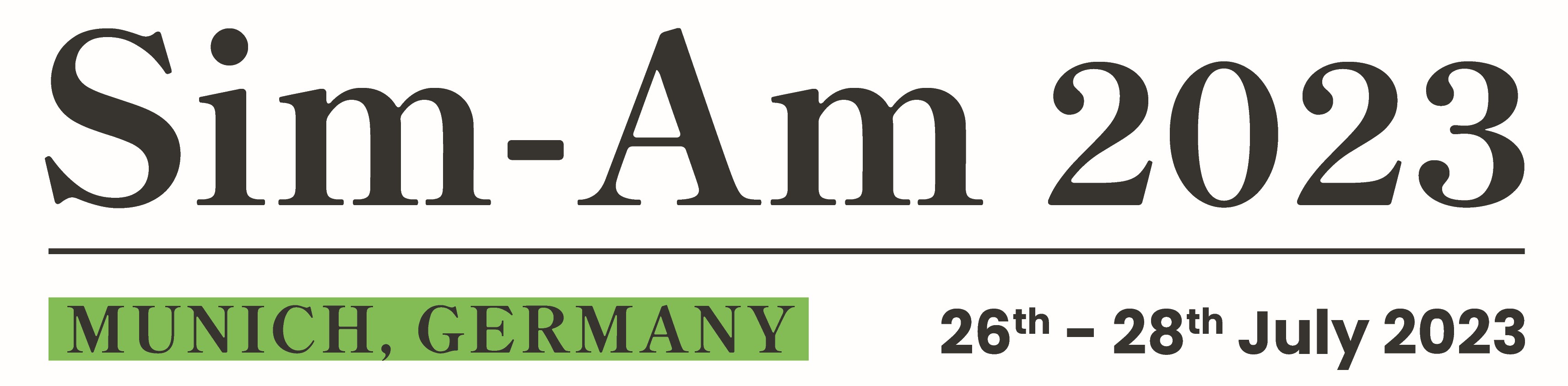
Scientific Machine Learning for Affordable High-Fidelity Simulations of Metal Additive Manufacturing
Please login to view abstract download link
Metal additive manufacturing (MAM) has received significant attention in recent years due to its significant advantages such as increased design flexibility for complex geometries, shorter production-cycle, and efficient use of raw materials. To fully realize the potential of MAM in the context of Industry 4.0, it is necessary to address challenges related to the mechanical reliability of printed parts and their associated costs. Currently, trial-and-error methods are the most common way of optimizing MAM process conditions for achieving the desired printing quality. Meanwhile, numerical simulations can provide a more profound understanding of the physical phenomena involved in the build process, leading to a more systematic optimization of process conditions, and ultimately making the `first-time-right' high-quality production possible. Achieving a thorough quantitative understanding of the process requires insights from models covering various physical aspects including thermal, mechanical, metallurgical, and fluid-dynamics interactions. However, high-fidelity simulations of such models are accompanied by significant computational costs and therefore have limits in applications, particularly in sensitivity and optimization analyses where solutions for a wide range of scenarios are required. To address this challenge, we initiated a project in 2021, with the support of the Swiss National Science Foundation (SNSF), to explore the feasibility of meaningful acceleration of these simulations without significant compromise in accuracy and reliability. Specifically, the project aims to develop solutions for thermal, microstructure, and residual stress simulations for the laser powder bed fusion (LPBF) process. To generate experimental validation data, Hastelloy X serve as the 'model material'. An overview of the results obtained so far, focusing on thermal and microstructure simulations, are presented. Several techniques have been examined to reduce the computational cost of thermal simulation for LPBF, including a multi-scale simulation strategy, surrogate modelling, and physics-informed neural networks (PINNs), where the advantages and limitations of each approach are discussed. In the field of microstructure modelling, a 'Neural Cellular Automata' method has been developed, which outperforms the conventional Cellular Automata with up to 6 orders of magnitude acceleration in computation speed.