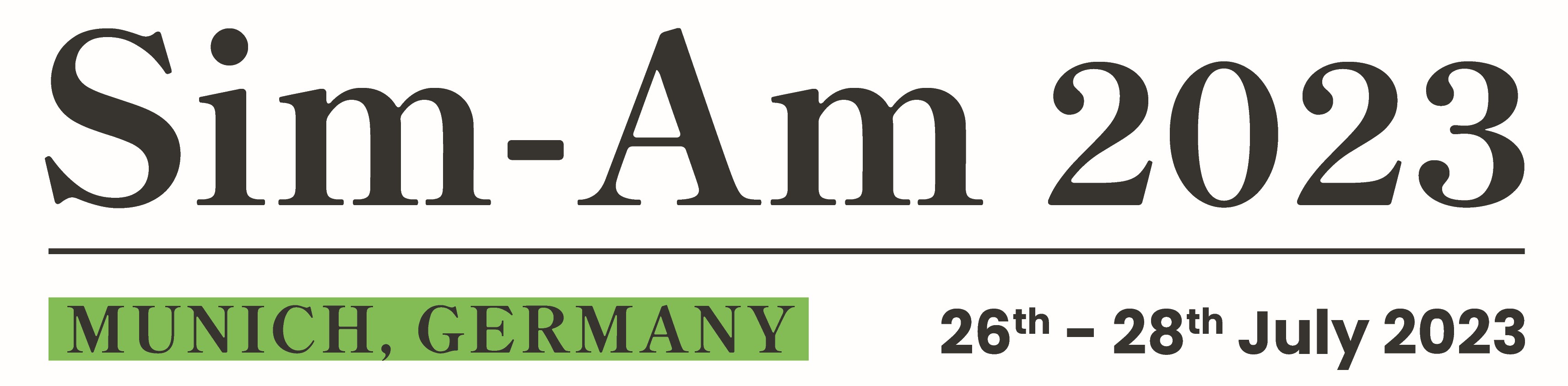
Validating High-Fidelity Computational Modeling of Metal Laser Powder Bed Fusion Additive Manufacturing Process with NIST AM Bench 2022
Please login to view abstract download link
Challenge 3 of the NIST 2022 Additive Manufacturing (AM) Benchmark carried out experimental measurements on bare metal plates of IN718. Laser power, scan speed, and spot diameter were varied in a set of seven conditions for single-track scans. Corresponding measurements for these seven conditions were made solid and liquid cooling rates, time above melting, and geometric measurements of the melt pool. In addition, multiple-track scanning was also made on the bare metal plate for the base settings. A calibrated cylindrical heat source for a finite volume based (FVM) C++ thermal CFD solver was utilized to carry out computational measurements of the test conditions. A heuristic calibration scheme maintaining a proportional relationship with volumetric energy density was used to predict the measurements completed by NIST with acceptable accuracy. However, it will be demonstrated that using a scaling law-based calibration scheme improves the prediction accuracy for the cases where a keyhole formation is expected. Further improving the calibration scheme to follow the physics-guided geometric constraints of the melt pool evolution demonstrates the highest fidelity compared to the first two methods. The physics-guided calibration scheme can capture the change in the melt pool geometry's aspect ratio and predict the measurements with higher accuracy. The developed method is then extended to predict the measurements for multiple-track scanning, and a quantitative comparison between the experiment and simulation is presented. Further improving upon the capabilities of the thermal CFD solver to predict the surface roughness and lack of fusion porosity of metal AM, a stochastic modeling and calibration strategy is proposed in which Monte Carlo Markov Chain (MCMC) sampling is used for statistical simulation. The Kullback-Leibler Divergence scheme is introduced to calibrate the cylindrical heat source against a statistically varying single-track measurement. Once calibrated, the same heat source is then used to solve an AM thin wall built to quantify the surface roughness and porosity. The results are compared against AFRL experiments to validate the process and the computational tool.