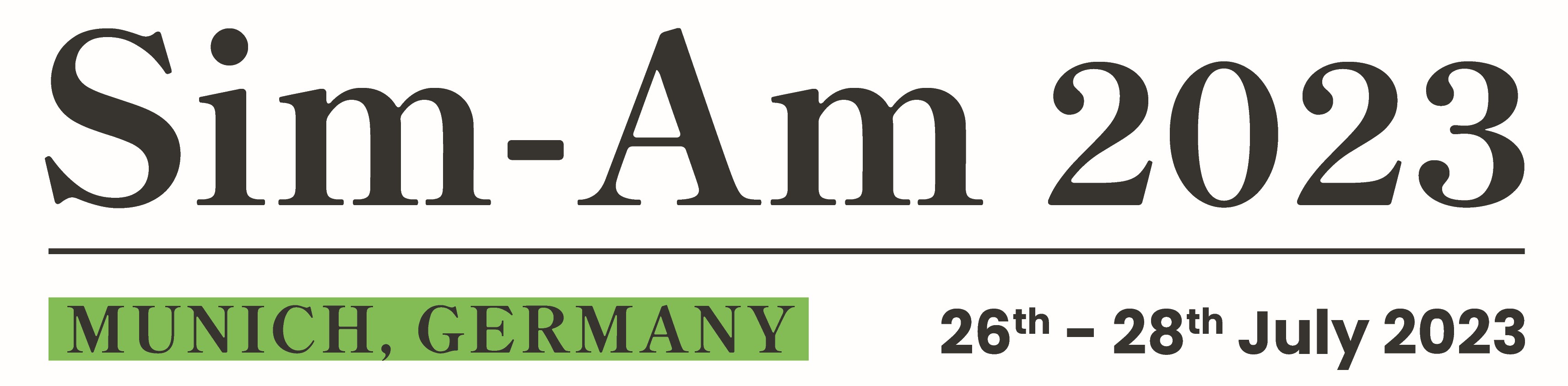
Temperature Prediction in Powder Bed Fusion Process using DeepONet
Please login to view abstract download link
Consistent simulation of additive manufacturing processes using standard and classic solvers requires very fine time steps and high spatial resolutions, which can lead to significant computational demands. These constraints might severely restrict the optimization and sensitivity analyses of the process in which many scenarios are to be considered [1]. Machine learning algorithms and data-driven methods have become increasingly popular in scientific computing due to their ability to provide fast and reasonably accurate solutions. Among these techniques, DeepONet [2] has emerged as a powerful tool for learning non-linear and complex operators, with smaller generalization errors compared to other well-known architectures. With appropriate training, DeepONet can effectively serve as a solution operator for parametric partial differential equations (PDEs). We utilize DeepONet to infer a real-time and consistent temperature field in the powder bed fusion (PBF) processes. The network is trained with different heat source paths as well as the heat source powers. We study how consistent our network would be for unseen scenarios. As a purely data-driven approach, one major drawback of DeepONet is that it requires lots of labeled data for supervised learning. In many engineering applications like PBF simulations, obtaining several training data from expensive numerical solvers would not be that convenient. In order to improve the accuracy and reduce the generalization errors, even without previous solutions, a physics-informed DeepONet is developed in which the training is performed in an unsupervised manner. References [1] Zhu, Q., Liu, Z., Yan, J. ”Machine learning for metal additive manufacturing: predicting temperature and melt pool fluid dynamics using physics-informed neural networks”, Comput Mech 67, 619–635 (2021). doi.org/10.1007/s00466-020-01952-9. [2] Lu, L., Jin, P., Pang, G. et al. ”Learning nonlinear operators via DeepONet based on the universal approximation theorem of operators”, Nat Mach Intell 3, 218–229 (2021). doi.org/10.1038/s42256-021-00302-5.