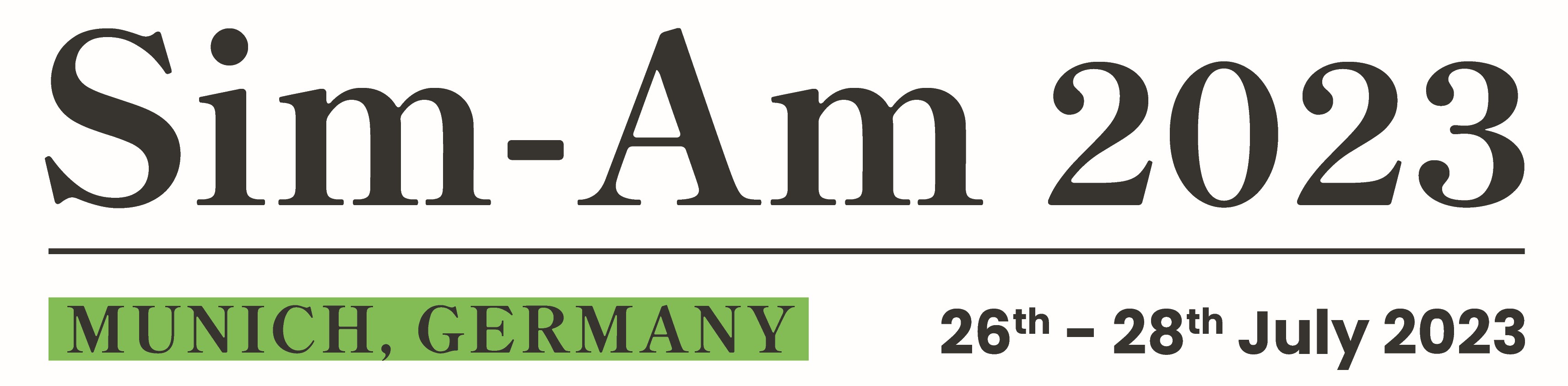
A data-driven framework for supporting experts with explainable process-structure linkages in metal additive manufacturing
Please login to view abstract download link
In recent years, the additive manufacturing (AM) community could witness a substantial rise of machine learning (ML) applications in AM. Often the used approaches resemble a black-box to experts, where trained models predict outputs without further information how the model based its decision-making-process. In the field of explainable artificial intelligence (XAI), the concept of Shapley values allows to derive additional information on the importance of input features on the model output. Applying this concept to metal AM, we present an open-access framework to identify process-microstructure linkages. The dataset used is from the physics-based kinetic Monte Carlo (kMC) method, which generates a total of 960 3D microstructures for powder-based solidification. Extracted directional grain size distributions to capture the morphology of the microstructure serve as target variables for training surrogate regression models. Applying Shapley analysis on the trained model directly correlates the importance of for example scanning strategy or the melt pool dimensions to morphological aspects of the as-built microstructure. Such correlations can be drawn on the local level for an individual process parameter setting, pairwise for pairs of process parameter changes, or globally to derive trends towards the whole dataset. Furthermore, the proposed approach navigates experts in the high-dimensional process parameter space to reach desired AM microstructures. The proposed approach can be seen as a ready-to-use methodology on finding and explaining process-microstructure linkages instead of providing a highly-accurate physical model.