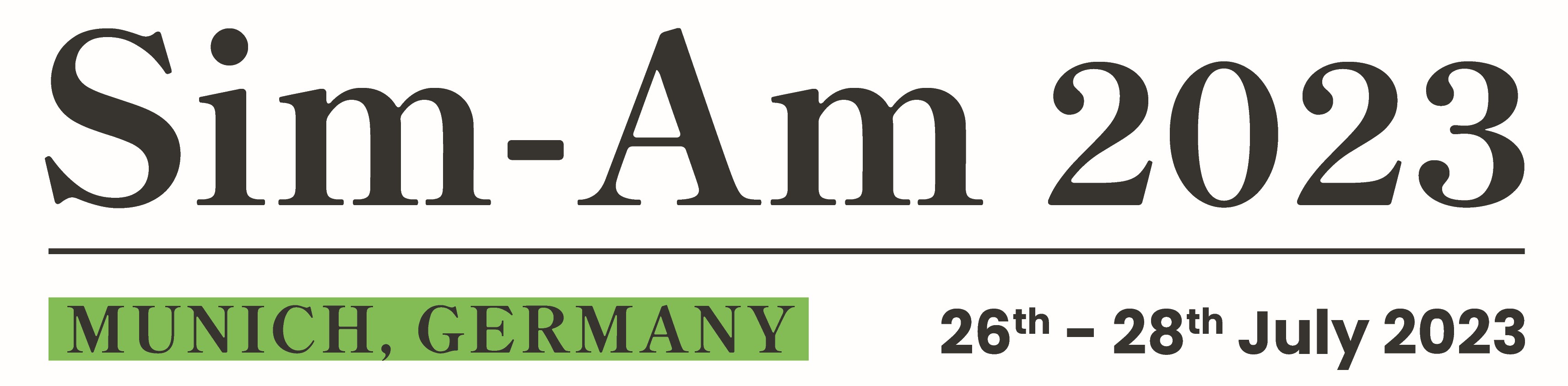
Integrating Physics-Informed Neural Networks with Cellular Automata for Microstructure Simulation of LPBF Hastelloy X
Please login to view abstract download link
Laser Powder Bed Fusion (LPBF) has enormous potential for additive manufacturing, but exploiting its full potential requires a deep understanding of the process-microstructure relationship to optimize process conditions and achieve the desired microstructure and properties in fabricated builds. Experimental exploration for deriving a quantitative description of this relationship is often time-consuming and costly. Numerical simulations offer a more efficient alternative, but their computational cost limits their application for optimization and inverse problems. To address this challenge, we present a reliable and computationally efficient tool for predicting the microstructure of LPBF Hastelloy X under varying process parameters by integrating physics-informed neural networks with cellular automata. The high computational efficiency of the developed framework enables us to solve the high-dimensional inverse problem of determining the unknown model parameters by using observations from a dedicated set of single-track LPBF depositions. Our findings demonstrate the potential of integrating scientific machine learning algorithms into numerical simulation frameworks for additive manufacturing and moving towards providing real-time simulation solutions for the control and optimization of additive manufacturing processes.