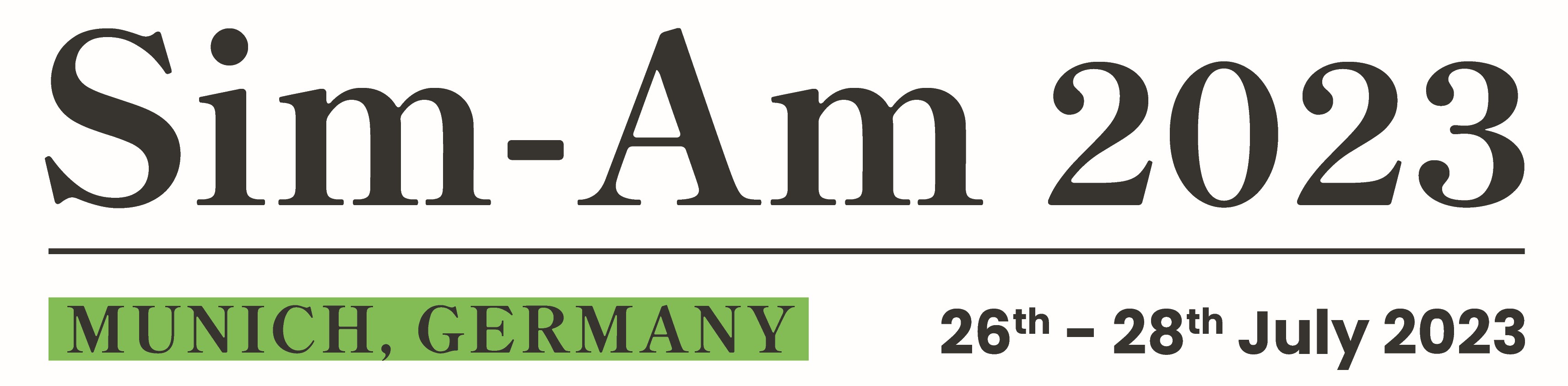
Design Digital Twins Enable Sustainable Manufacturing in Powder-bed Fusion and Directed Energy Deposition Additive Manufacturing Processes
Please login to view abstract download link
Digital process development for additive manufacturing (AM) is emerging as a sustainable, cost-attractive alternative to the traditional experimental trial-and-error approach whereby computer modeling and simulations are used to explore the process window for a given material, 3D printer, and part. At the same time, digitalization is a hard task requiring validated, high-fidelity models at different length scales (part and melt pool) and time scales (solidification to build time). Such challenges are equally present in powder-bed fusion (PBF) as well as in directed energy deposition (DED) AM. Here, we report on our efforts to develop a design digital twin for the PBF and DED processes by developing a fully in-house integrated model including thermal simulation at the scale of the part, classification of thermal history to identify key locations in the component, melt-pool model which resolves porosity and microstructure explicitly, mean-field crystal plasticity model to calculate mechanical properties, metallurgical phase fraction model, and residual stress and distortion at part scale. By deploying such design twins, users are able to explore the role of process parameters in part quality digitally rather than experimentally, therefore reducing the need for physical feedstock material and machine time.